Spark Stage 源码分析
# 1. Stage 概述
Spark 会将一个 Job 划分为多个 Stage,划分的原则是每遇到一个宽依赖就会进行一次划分,Stage 内部的 RDD 都是窄依赖。这样做的好处是可以让有依赖关系的 Stage 顺序执行,没有依赖关系之间并行执行。同时每一个 Stage 内部的 RDD Task 是可以并行执行的。
具有窄依赖关系的 RDD 操作(如:map、fileter 等)在每个 Stage 中以 TaskSet 流水线的方式执行,但是具有宽依赖关系的操作(如:groupBy、join 等)需要多个 Stage(一个 Stage 输出文件,另一个 Stage 之后读取这些文件)。最后,Stage 之间是通过宽依赖连接的,但是在 Stage 内部的计算是并行执行的,而真正执行计算的是各个 RDD 的 compute()
函数。
Stage 也是有重试机制的,当一个 Stage 执行失败之后,会被重新拉起执行。
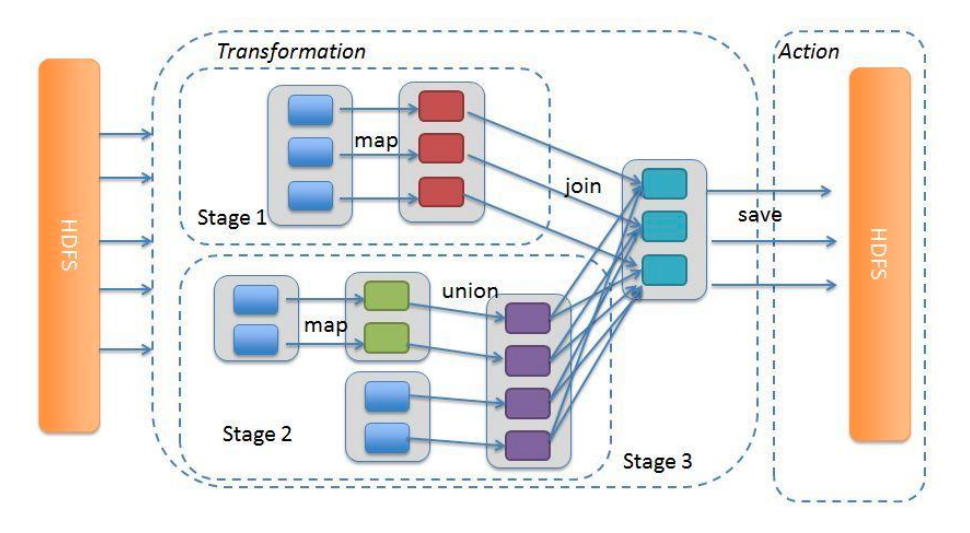
# 2. 源码
抽象类Stage
有两个实现类,分别是ShuffleMapStage
和ResultStage
。ResultStage
是最后一个执行的 Stage,其它所有 Stage 都是ShuffleMapStage
。
ShuffleMapStage
是执行 DAG 中的中间阶段,在 shuffle 之前执行,为 shuffle 生成数据。执行过程中会将 map 端的输出进行保存,稍后 reduce 阶段就会获取 map 端的输出。ShuffleMapStage
中的算子都是 Transform 算子。ResultStages
在 RDD 的某些分区上应用函数来计算操作的结果,ResultStages
中的算子是 Action 算子。。
# 2.1 Stage 源码
private[scheduler] abstract class Stage(
val id: Int, // Stage id
val rdd: RDD[_], // 当前 Stage 所包含的 RDD。对于 ShuffleMapStage 指的是执行 map 任务的 RDD,对于 ResultStage 指的是执行 action 的RDD。
val numTasks: Int, // 当前 Stage 所包含的 task 数量
val parents: List[Stage], // 当前 Stage 所包含的父 Stage 列表
val firstJobId: Int, // 第一个提交当前 Stage 的 Job id。因为一个 Stage 可以被多个 Job 使用,当采用 FIFO 调度时,通过 firstJobId 首先计算来自较早 Job 的 Stage,可以帮助在故障时更快恢复。
val callSite: CallSite // 应用程序中与当前 Stage 相关的调用栈信息
)
extends Logging {
// 当前 Stage 的分区数量
val numPartitions = rdd.partitions.length
// 当前 Stage 所属的 Job id 集合,说明一个 Stage 可以被多个 Job 使用。
val jobIds = new HashSet[Int]
// 下次尝试 Stage 的 id
private var nextAttemptId: Int = 0
val name: String = callSite.shortForm
val details: String = callSite.longForm
// 最近一次尝试的 Stage 信息
private var _latestInfo: StageInfo = StageInfo.fromStage(this, nextAttemptId)
// 失败的 Stage 尝试 id 的集合,为了避免同一个尝试多次执行以及无限制重试
val failedAttemptIds = new HashSet[Int]
private[scheduler] def clearFailures() : Unit = {
failedAttemptIds.clear()
}
// 创建新的 Stage 尝试
def makeNewStageAttempt(
numPartitionsToCompute: Int,
taskLocalityPreferences: Seq[Seq[TaskLocation]] = Seq.empty): Unit = {
val metrics = new TaskMetrics
metrics.register(rdd.sparkContext)
// 创建新的尝试
_latestInfo = StageInfo.fromStage(
this, nextAttemptId, Some(numPartitionsToCompute), metrics, taskLocalityPreferences)
// 修改尝试 id
nextAttemptId += 1
}
def latestInfo: StageInfo = _latestInfo
override final def hashCode(): Int = id
override final def equals(other: Any): Boolean = other match {
case stage: Stage => stage != null && stage.id == id
case _ => false
}
// 寻找未执行完的分区,需要子类实现
def findMissingPartitions(): Seq[Int]
def isIndeterminate: Boolean = {
rdd.outputDeterministicLevel == DeterministicLevel.INDETERMINATE
}
}
1
2
3
4
5
6
7
8
9
10
11
12
13
14
15
16
17
18
19
20
21
22
23
24
25
26
27
28
29
30
31
32
33
34
35
36
37
38
39
40
41
42
43
44
45
46
47
48
49
50
51
52
53
54
55
56
57
58
59
60
61
2
3
4
5
6
7
8
9
10
11
12
13
14
15
16
17
18
19
20
21
22
23
24
25
26
27
28
29
30
31
32
33
34
35
36
37
38
39
40
41
42
43
44
45
46
47
48
49
50
51
52
53
54
55
56
57
58
59
60
61
# 2.2 ShuffleMapStage 源码
private[spark] class ShuffleMapStage(
id: Int, // Stage id
rdd: RDD[_], // 当前 Stage 所包含的 RDD。对于 ShuffleMapStage 指的是执行 map 任务的 RDD,对于 ResultStage 指的是执行
numTasks: Int, // 当前 Stage 所包含的 task 数量
parents: List[Stage], // 当前 Stage 所包含的父 Stage 列表
firstJobId: Int, // 第一个提交当前 Stage 的 Job id。因为一个 Stage 可以被多个 Job 使用,当采用 FIFO 调度时,通过 firstJobId 首先计算来自较早 Job 的 Stage,可以帮助在故障时更快恢复。
callSite: CallSite, // 应用程序中与当前 Stage 相关的调用栈信息
val shuffleDep: ShuffleDependency[_, _, _], // 对应的依赖关系
mapOutputTrackerMaster: MapOutputTrackerMaster)
extends Stage(id, rdd, numTasks, parents, firstJobId, callSite) {
// 与此 Stage 相关联的 ActiveJob 列表
private[this] var _mapStageJobs: List[ActiveJob] = Nil
// 未计算的分区或在已丢失的 executor 上计算的分区集合,DAGScheduler 会根据此属性判断 Stage 何时完成。
val pendingPartitions = new HashSet[Int]
override def toString: String = "ShuffleMapStage " + id
def mapStageJobs: Seq[ActiveJob] = _mapStageJobs
def addActiveJob(job: ActiveJob): Unit = {
_mapStageJobs = job :: _mapStageJobs
}
def removeActiveJob(job: ActiveJob): Unit = {
_mapStageJobs = _mapStageJobs.filter(_ != job)
}
def numAvailableOutputs: Int = mapOutputTrackerMaster.getNumAvailableOutputs(shuffleDep.shuffleId)
def isAvailable: Boolean = numAvailableOutputs == numPartitions
override def findMissingPartitions(): Seq[Int] = {
mapOutputTrackerMaster
.findMissingPartitions(shuffleDep.shuffleId)
.getOrElse(0 until numPartitions)
}
}
1
2
3
4
5
6
7
8
9
10
11
12
13
14
15
16
17
18
19
20
21
22
23
24
25
26
27
28
29
30
31
32
33
34
35
36
37
38
39
2
3
4
5
6
7
8
9
10
11
12
13
14
15
16
17
18
19
20
21
22
23
24
25
26
27
28
29
30
31
32
33
34
35
36
37
38
39
# 2.3 ResultStage 源码
private[spark] class ResultStage(
id: Int, // Stage id
rdd: RDD[_], // 当前 Stage 所包含的 RDD。对于 ShuffleMapStage 指的是执行 map 任务的 RDD,对于 ResultStage 指的是执行
val func: (TaskContext, Iterator[_]) => _, // 对 RDD 执行计算的函数
val partitions: Array[Int], // RDD 各个分区索引组成的数组
parents: List[Stage], // 当前 Stage 所包含的父 Stage 列表
firstJobId: Int, // 第一个提交当前 Stage 的 Job id。因为一个 Stage 可以被多个 Job 使用,当采用 FIFO 调度时,通过 firstJobId 首先计算来自较早 Job 的 Stage,可以帮助在故障时更快恢复。
callSite: CallSite // 应用程序中与当前 Stage 相关的调用栈信息
)
extends Stage(id, rdd, partitions.length, parents, firstJobId, callSite) {
private[this] var _activeJob: Option[ActiveJob] = None
def activeJob: Option[ActiveJob] = _activeJob
def setActiveJob(job: ActiveJob): Unit = {
_activeJob = Option(job)
}
def removeActiveJob(): Unit = {
_activeJob = None
}
override def findMissingPartitions(): Seq[Int] = {
val job = activeJob.get
(0 until job.numPartitions).filter(id => !job.finished(id))
}
override def toString: String = "ResultStage " + id
}
1
2
3
4
5
6
7
8
9
10
11
12
13
14
15
16
17
18
19
20
21
22
23
24
25
26
27
28
29
30
2
3
4
5
6
7
8
9
10
11
12
13
14
15
16
17
18
19
20
21
22
23
24
25
26
27
28
29
30
上次更新: 2023/11/07, 07:39:51